In the domain of meteorology, weather forecasting has always been a complex and intricate discipline. The introduction of an innovative model, GraphCast, developed by Google DeepMind, is set to significantly enhance the accuracy and speed of these forecasts. Leveraging the power of Artificial Intelligence (AI), GraphCast has been designed to provide highly precise 10-day weather predictions in less than a minute.
1. The Advent of GraphCast: A New Era in Weather Forecasting
Weather predictions have always played a pivotal role in our daily lives, from planning our day-to-day activities to preparing for natural disasters. With the introduction of GraphCast, the landscape of weather forecasting is set to undergo a significant transformation. This revolutionary AI model outperforms traditional weather forecasting systems and even other AI-based models in terms of speed and accuracy.
1.1 The Need for Improved Weather Forecasting
Understanding the weather has always held paramount importance for human civilization. It influences our daily routines, clothing choices, travel plans, agricultural practices, and more. In the face of increasingly extreme weather conditions due to climate change, the need for swift and accurate weather forecasts has never been more critical.
1.2 The Role of AI in Weather Forecasting
The integration of AI into weather forecasting is ushering in a new era of meteorological predictions. GraphCast, an AI model developed by Google DeepMind, is a testament to this advancement. Leveraging machine learning, this model can make medium-range weather forecasts with unprecedented accuracy. It is capable of predicting weather conditions up to 10 days in advance, faster and more accurately than the industry’s gold-standard weather simulation system.
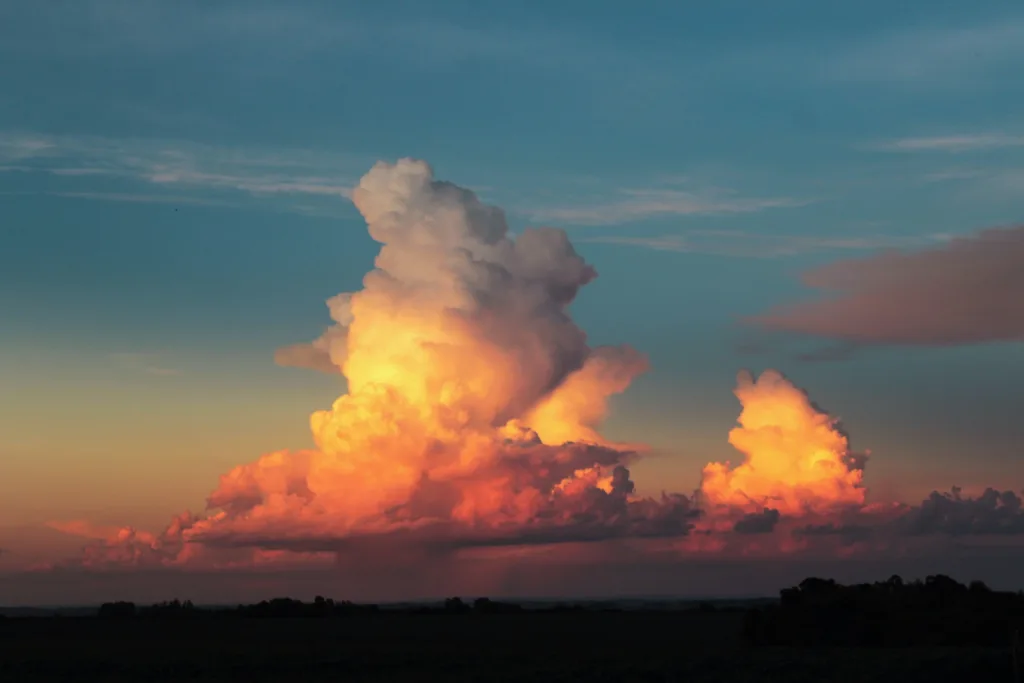
2. Understanding Google DeepMind’s GraphCast
GraphCast functions as an AI-weather forecasting system, using machine learning and Graph Neural Networks (GNNs) to process spatially structured data. It functions on a high resolution of 0.25 degrees longitude/latitude, which equates to more than a million grid points covering the Earth’s surface.
2.1 The Working Principle of GraphCast
The underpinning principle of GraphCast is grounded in machine learning, where forecast models are trained from historical data. This approach, known as Machine Learning-based Weather Prediction (MLWP), facilitates the improvement of forecast accuracy by identifying patterns in the data that cannot be easily represented in explicit equations.
2.2 The Efficiency of GraphCast
GraphCast’s working model is impressively efficient. While its training process is computationally intensive, the resulting forecasting model is highly effective. A 10-day forecast with GraphCast takes less than a minute on a single Google TPU v4 machine. In contrast, conventional approaches like the High RESolution forecast (HRES) can take hours of computation on a supercomputer with hundreds of machines.
3. The Superior Performance of GraphCast
The performance of GraphCast has been evaluated against the gold-standard deterministic system, HRES. GraphCast consistently provided more accurate predictions on over 90% of the tested variables and forecast lead times.
3.1 Assessing the Accuracy of GraphCast
In medium-range weather forecasting, predicting atmospheric variables up to 10 days ahead, traditional NWP-based systems are considered the most accurate. However, GraphCast has shown a remarkable ability to rival, and in many cases surpass, these traditional systems in terms of accuracy.
3.2 GraphCast in Real-World Scenarios
GraphCast’s performance has been evaluated thoroughly for real-world applications. For instance, the model was tested against the European Centre for Medium-Range Weather Forecasts’ gold-standard system, known as the High RESolution forecast (HRES). The testing revealed that GraphCast was more spot-on in its predictions by a staggering 99.7% in some cases.
4. The Implications of GraphCast for Extreme Weather Events
One of the notable features of GraphCast is its ability to predict extreme weather events with remarkable accuracy. Despite not being specifically trained to identify severe weather conditions, GraphCast has proven effective in anticipating cyclone movements, atmospheric rivers, and extreme temperatures.
4.1 Predicting Tropical Cyclone Tracks
Improving the accuracy of tropical cyclone tracking can help avert injury and loss of life, as well as reduce economic harm. GraphCast has proven to be effective in predicting cyclone movement more accurately than traditional models.
4.2 Characterizing Atmospheric Rivers
Atmospheric rivers are narrow regions of the atmosphere that are responsible for the majority of the poleward water vapor transport across the mid-latitudes. GraphCast can effectively characterize atmospheric rivers, which can be crucial for preparing emergency responses together with AI models to forecast floods.
4.3 Predicting Extreme Temperatures
Extreme temperatures, both hot and cold, can be dangerous and disrupt human activities. GraphCast has shown its effectiveness in predicting events above the top 2% climatology across location, time of day, and month of the year.
5. The Future of AI in Weather Forecasting
With the introduction of GraphCast, AI-based weather forecasting is poised to benefit billions of people in their everyday lives. As weather patterns evolve in a changing climate, GraphCast will evolve and improve as higher quality data becomes available.
5.1 Open Sourcing the GraphCast Model
To make AI-powered weather forecasting more accessible, the creators of GraphCast have open-sourced their model’s code. This move is anticipated to unlock a range of possibilities for researchers, from tailoring the model for particular weather phenomena to optimizing it for different parts of the world.
5.2 GraphCast and Other Weather Prediction Systems
GraphCast is part of a suite of state-of-the-art weather prediction systems from Google DeepMind and Google Research. These include a regional Nowcasting model that produces forecasts up to 90 minutes ahead, and MetNet-3, a regional weather forecasting model already in operation across the US and Europe that produces more accurate 24-hour forecasts than any other system.
With the advent of GraphCast, the domain of weather forecasting is set to undergo a significant transformation. As the model continues to evolve and improve, it promises to provide increasingly accurate and efficient forecasts, benefiting billions of people in their everyday lives. This ground-breaking innovation in weather forecasting is just the tip of the iceberg when it comes to the potential applications of AI in understanding broader patterns of our climate.